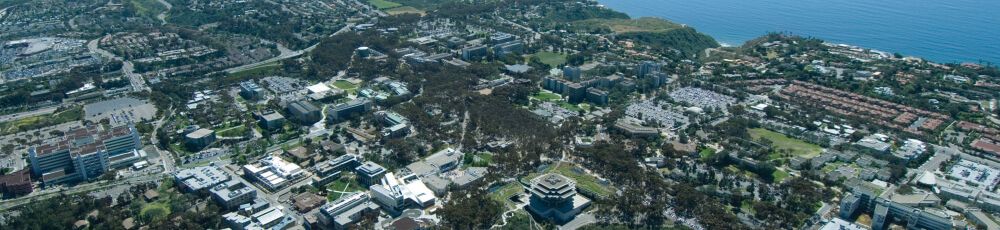
Credit: UC San Diego Publications | Copyright Regents of the University of California
Research Interests
I am broadly interested in computational methods and numerical analysis for control, optimization, design and uncertainty quantification of complex and large-scale systems. I work on using reduced-order models in the context of multifidelity and data-driven modeling, optimization and control, uncertainty quantification, reliability-based design, and design under uncertainty. I am affiliated with the Center for Extreme Events Research (CEER) as well as the Center for Computational Mathematics (CCoM).
Contact
Boris Krämer
Associate Professor
Department of Mechanical and Aerospace Engineering
University of California San Diego
Jacobs Hall (EBU1) | Room 4209
9500 Gilman Drive | La Jolla | CA 92093-0411
- +1 (858) 246-5327
- bmkramer at ucsd dot edu
- https://www.linkedin.com/in/kramerboris
- Google Scholar: Papers and citations
- ResearchGate: Published research and discussions
- ORCiD: Persistant digital identifier
-
Publons: Peer-reviewing profile
Upcoming events
July 15-18, 2025: I will be presenting recent work (with Nick Corbin) titled "The polynomial-polynomial regulator for high dimensional nonlinear control" at the 16th Viennese Conference on Optimal Control and Dynamic Games in Vienna, Austria.
July 20-24, 2025: Graduate student Hyeonghun Kim will be presenting his work " Physically consistent predictive reduced-order modeling by enhancing operator inference with state constraints" at the 18th National Congress on Computational Mechanics (USNCCM) in Chicago.
July 28-30, 2025: The SIAM Conference on Control and Its Applications (CT25) is co-hosted with the SIAM Annual Meeting (AN25) in Montreal, Canada. Stay tuned for updates. My control-oriented talk is on Monday July 28, 4:00pm about "Data-driven reduced-order models for control of soft robots" in MS9 Data-driven Model Reduction for Control (thanks to Peter Benner and Tobias Breiten for the invitation). I will also give a talk at SIAM AN25 "Towards controllable and observable reduced-order models for digital twins" (thanks to Harbir Antil for the invitation).
August 11-15, 2025: I'll be attending and presenting at the workshop Mathematical and Computational Foundations of Digital Twins at the Centre International de Rencontres Mathématiques (CIRM) in Luminy, France.
August 24, 2025: Fourth-year graduate student Nick Corbin will be giving a talk on "Feedback Control of Nonlinear Systems Using The Polynomial-Polynomial Regulator" as part of the PDE control workshop at the 9th IEEE Conference on Control Technology and Applications (CCTA) here in San Diego.
September 22-26, 2025: What an honor that 5th-year graduate student Opal Issan will be in invited plenary speaker at the 3rd Machine Learning in Heliophysics Conference in Madrid, Spain.
Special Issue Alert:: I serve on the editorial boards of two special issues and we are inviting high-quality research contributions to both. The Model Reduction and Surrogate Modeling 2024 (MORe24) collection has two participating journals, Advances in Computional Mathmematics (ACOM) and Computational Science and Engineering (CSE) and a deadline of June 30, 2025. This topical collection is reflects the research and discussions from the second Model Reduction and Surrogate Modeling (MORE) Conference held in September 2024 in San Diego. The other Special Issue on Reduced Order Modeling, Generative AI, and SciML in Digital Twins will be in Structural and Multidisciplinary Optimization (SAMO) with submission deadline July 31, 2025.
Recent News
June 23-27, 2025: This was a busy week for our group! Graduate student Jaime Landeros attended the Solar Heliospheric and INterplanetary Environment (SHINE) in Charleston, South Carolina, where he presented his work on data analysis and uncertainty quantification for coronal hole boundary detection in multi-spectral solar imagery. Graduate student Albani Olivieri participated in the EaGR: Early graduate research in applied mathematics workshop at the American Institute of Mathematics, Pasadena, CA. Graduate student Opal Issan presented a poster on understanding secondary drift-driven instabilities caused by whistler waves using quasilinear theory at the 2025 Geospace Environment Modeling (GEM) Workshop in Des Moines, Iowa.
June 16-25, 2025: Graduate students Hyeonghun Kim and Justin Burzachiello attended the Summer School and Hackathon event on Structure-Preserving Scientific Computing and Machine Learning at the University of Washington. Thanks to NSF and the organizers for their support of Justin and Jaime.
June 15-18, 2025: I presented joint work with Postdoc Yulin Guo on "Optimization under uncertainty for efficient laser powder-bed fusion manufacturing" at the 6th International Conference on Uncertainty Quantification in Computational Science and Engineering (UNCECOMP) in Rhodes, Greece.
June 6, 2025: Congratulations to graduate student Nate Linden for defending his PhD thesis "Uncertainty quantification methods improve mechanistic predictability in systems biology". Nate joined UCSD (co-advised with Prof. Padmini Rangamani) in 2020 from the University of Washington with degrees in Bioengineering and Applied Mathematics, and hasn't looked back. He fully embraced the interdisciplinary nature of the research at the intersection of uncertainty quantification and systems biology and wrote several nice papers along the way.
June 1-6, 2025: Postdoc Yulin Guo presented his work on risk-based design optimization for powder bed fusion metal additive manufacturing at the 14th International Conference on Structural Safety and Reliability - ICOSSAR'25 hosted by the University of Southern California.
June 1, 2025: Congratulations to 4th-year graduate student Opal Issan for winning the 2024-2025 Outstanding PhD Student of the Year Award in the Department of Mechanical and Aerospace Engineering and also to 4th-year graduate student Nick Corbin for winning the 2024-2025 Outstanding TA award for his excellent work in the 143B (Linear Controls) class.
May 1, 2025: SIAM summarized our Forward-looking Panel at CSE25 in a nice blog post. Check it out!
April 16-18, 2025: Postdoc Yulin Guo presented his work "Optimization under Uncertainty for Efficient Laser Powder Bed Fusion Manufacturing" at the East Cost Optimization Meeting 2025 at George Mason University. Thanks to the organizers for arranging travel support and putting together this nice conference.
April 12, 2025: Our group once again participated in the Barrio Logan Science and Art Expo with a science booth on solar wind phenomena, and partnering up with the undergraduate SEDS and RPL clubs at UCSD. Touching young kids and their imagination is always such a great experience.
April 4, 2025: Graduate student Hyeonghun Kim co-authored Parametric Operator Inference to Simulate the Purging Process in Semiconductor Manufacturing, led by Dr. Seunghyon Kang. Dr. Kang spent a year-long sabbatical from Samsung Electronics Co., Ltd at our group from March 2024-March 2025. His work at Samsung is mainly on process control monitoring, and he was interested in leverating reduced-order modeling to speed up that process. During that year, we worked on data-driven ROMs in the parametric regime via Operator Inference. The CFD simulations had 1.25M degrees of freedom, and he was able to significantly speed up the analysis. We are looking forward to working with Dr. Kang to integrate into Samsung's processes.
March 6, 2025: Postdoc Harsh Sharma posted his article on Nonlinear energy-preserving model reduction with lifting transformations that quadratize the energy to arxiv. In this article, we continue our work on finding auxiliary lifting variables to rewrite non-polynomial systems in quadratic form (doing so exactly, without approximation), this time with a constraint on the transformation so that the lifted system remains energy conserving.
March 3-7, 2025: It was very nice to once again be at the SIAM Conference on Computational Science and Engineering (CSE25) in Fort Worth, TX, where I got to meet old friends and met new colleauges and learn about so many great research directions. From our group, Postdoc Harsh Sharma presented our work on Structure-Preserving Model Reduction of Conservative Pdes Via Lifting Transformations, 5th-year graduate student Nate Linden presented his work (joint with Padmini Rangamani) on Bayesian Multimodel Inference to Account for Model Uncertainty in Systems Biology, 2nd year graduate student Steven Nguyen presented his ongoing research on Data-Driven Control via Semidefinite Programming in Nonlinear Systems and I presented our work (led by 4th year graduate student Nick Corbin) on Nonlinear Balanced Truncation Model Reduction in High Dimensions.
February 24-25, 2025: As part of our MURI project on ``Mathematics of Digital Twins", we organized the Advances in Digital Twins workshop at Stanford University. This workshop brought together many experts from both academia, industry and federal agengies (NASA) to explore how digital twins are shaping the future.
February 7, 2025: Graduate student Hyeonghun Kim just posted his first journal article Physically consistent predictive reduced-order modeling by enhancing Operator Inference with state constraints to arxiv. In this work, we consider a biomass combustion problem and use Operator Inference to learn a quadratic reduced-order model (ROM). Originally, the models were unstable and predicted negative species. After many different attempts with regularization and stabilization schemes, we finally implemented species limiters (as commonly done in CFD) and not only did we get physically consistent predictions, but also long-term stability of the nonlinear model and very good species conservation properties---although more work needs to be done there.
January 14, 2025: Congratulations to 4th year graduate student Opal Isssan for having her fourth first-authored journal article since joining my group published. Her article/Short Note Conservative closures of the Vlasov-Poisson equations based on symmetrically weighted Hermite spectral expansion appeared in the Journal of Computational Physics. Therein, Opal derived conservative closures for the Vlasov-Poisson equations discretized in velocity via the symmetrically weighted Hermite spectral expansion. Through thorogh analysis, she showed thatno closure can simultaneously restore the conservation of mass, momentum, and energy in this formulation. The properties of the analytically derived conservative closures of each conserved quantity are validated numerically by simulating an electrostatic benchmark problem: the Langmuir wave. Both the numerical results and analytical analysis indicate that closure by truncation (i.e. setting the last Hermite moment to zero) is the most suitable conservative closure for the symmetrically weighted Hermite formulation.
January 6-10, 2025: Postdoc Harsh Sharma and I participated in the workshop Computational Learning for Model Reduction at the NSF-sponsored Institute for Computational and Experimental Research in Mathematics (ICERM) at Brown University. I gave a talk on "Structure-Preserving Learning of High-Dimensional Lagrangian and Hamiltonian Systems" and Harsh presented a poster on "Lagrangian operator inference enhanced with structure-preserving machine learning for nonintrusive model reduction of mechanical systems".
December 16-19, 2024: The 63rd IEEE Conference on Decision and Control was held in Milan. Fourth-year graduate student Nick Corbin presented his work "Computing Solutions to the Polynomial-Polynomial-Regulator Problem" in the invited session on "Estimation and Control of Distributed Parameter Systems" by Profs. Michael Demetriou and Weiwei Hu.
December 12, 2024: Fourth-year graduate student Nick Corbin gave a talk on Nonlinear Balanced Truncation Model Reduction at La Sapienza, Rome, hosted by Prof. Alessandro Alla and his group.
December 8-13, 2024: It was a fantastic experience to participate in the Mini-Workshop Data-driven Modeling, Analysis, and Control of Dynamical Systems at the Mathematisches Forschungsinstitut Oberwolfach in the beautiful Black Forest. Thanks to Karl Worthmann, Claudia Schillings and Clancy Rowley for the organization. The black-board talks, randomized seating for meals and overall Oberwolfach experience is as stimulating of a research environment as there is.
November 7, 2024: Congratulations to 4th year graduate student Nick Corbin for having his first journal article Scalable Computation of H-infinity Energy Functions for Polynomial Control-Affine Systems appear in IEEE Transactions on Automatic Control. In this work, we present a scalable approach to computing nonlinear balancing energy functions for control-affine systems with polynomial nonlinearities. Al'brekht's power-series method is used to solve the Hamilton-Jacobi-Bellman equations for polynomial approximations to the energy functions. The contribution of this article lies in the numerical implementation of the method based on the Kronecker product, enabling scalability to over 1000 state dimensions, well above the current state of the art.
November 7-9, 2024: I attended the AAAI Fall Symposium on Integrated Approaches to Computational Scientific Discovery (Arlington, VA) where I'll give a talk on "Discovering Quadratic Representations of PDEs".
September, 2023: Welcome to our new graduate students! Albani Oliveiri joins us with an M.S. and B.S. in Computer Science Engineering from the Universidad de Chile in Santiago, where she worked on designing and developing a quadratization/lifting algorithm for PDE models. She will continue this work for the purpose of model reduction. Jaime Landeros is joining us with a B.S. in Aerospace Engineering at Cal Poly Pomona and a three-year internship with the NASA Goddard Solar Physics Laboratory where he explored physics-based surrogate modeling, uncertainty quantification, and data analysis in space weather and liquid propulsion applications. He will be working on data-driven model reduction for quantifying the uncertainties in global-scale, multi-physics models of space weather.
September 25-27, 2024: I attended the 60th Annual Allerton Conference on Communication, Control, and Computing which will be held at the University of Illinois at Urbana-Champaign. There, I'll be giving an invited presentation on "Scalable computations for nonlinear balanced truncation model reduction" in the special session on Duality in Control, Inference, and Learning (organized by Prashant Mehta and Max Raginsky).
September 15-19, 2024: The DFG-funded Collaborative Research Center on Data Assimilation (SFB 1294) at the University of Potsdam, Germany organized the CRC International Summer School 2024 at the Lindner Hotels Boltenhagen. This 5-day summer school will brought together the PhD students and researchers from the CRC and several international research groups from UC San Diego and RIKEN (Japan). Three PhD students from our group, Nate Linden-Santangeli, Opal Issan and incoming PhD student Jaime Landeros joined the summer school. Thanks a lot to Professor Sebastian Reich for the invitation and the CRC for the support.
September 9-13, 2024: It was such an honor and a lot of fun to host the Model Reduction and Surrogate Modeling (MORE) Conference here at the Scripps Seaside Forum on the campus of the University of California San Diego. This 5-day conference brought together the international community of computational scientists, engineers, mathematicians, and domain experts from industry, the national laboratories and academia to address the topic of model reduction and surrogate modeling for high-dimensional complex systems. The five days went by fast, with lots of great talks and plenty of old and new faces in the model reduction community.
August 19-21, 2024: Postdoc Yulin Guo presented his work on "Sparsity-promoting Design under Uncertainty for a Char Combustion problem" at the 21st working conference of the IFIP Working Group 7.5 on Reliability and Optimization of Structural Systems in Berkeley, CA. The IFIP WG7.5 aims to promote research, develop theory and applications, disseminate and exchange information and encourage education on structural systems reliability and optimization. The conference is a forum for active discussion of topics related to structural systems reliability and optimization.
July 21-26, 2024: The 16th World Congress on Computational Mechanics happened in Vancouver. Two group members presented their work there, Postdoc Harsh Sharma as well as our former Postdoc, and now Assistant Professor, Dongjin Lee.
July 23-26, 2024: It was exciting to participate in the 2nd Joint Meeting of the Unione Matematica Italiana (UMI) and the American Mathematical Society (AMS) in Palermo, Italy, despite record temperatures! Together with Dr. Alessandro Alla (Università Ca Foscari Venezia) we organized a special session on "Exploiting Low-rank Structures for the Solution of PDEs", held on July 25.
July 20, 2024: Our article Bayesian identification of nonseparable Hamiltonians with multiplicative noise using deep learning and reduced-order modeling (with Nick Galioto and Alex Gorodetsky (University of Michigan)) appeared in CMAME. In this article we incorporate geometric structure into the Bayesian learning approach for stochastic systems, to truly account for the complexities of such models. For high-dimensional systems, we also add a model reduction step so the Bayesian learning algorithms can operate on low-dimensioanal data.
July 15, 2024: The workshop on Structural Priors as Inductive Biases for Learning Robot Dynamics is part of the 20th Robotics: Science and Systems (RSS) Conference in Delft, NL. It was great to meet people from different backgrounds, particularly robotics, trying to solve similar problems. As part of the workshop, I presented a poster on "Preserving Lagrangian Structure in Data-driven Reduced-order Modeling of Soft Robots".
July 10-12, 2024: The 2024 American Control Conference took place in Toronto. Third-year graduate student Nick Corbin presented his work " Scalable Computation of H-Infinity Energy Functions for Polynomial Drift Nonlinear Systems " in the invited session on "Estimation and Control of Distributed Parameter Systems" by Profs. Michael Demetriou and Weiwei Hu.
July 4, 2024: I am giving a talk at the seminar of the CRC 1173: Wave Phenomena (Department of Mathematics) at the Karlsruhe Institute of Technology (KIT), my undergraduate alma mater.
July 2-3, 2024: I am visiting the Institute of Engineering and Computational Mechanics at the University of Stuttgart (hosted by Prof. Joerg Fehr) where I'll be presenting about "Learning Lagrangian Models from High-dimensional Data".
July 1, 2024: Thank you to Dr. Henning Bonart for inviting me to give a talk at the Institute for Nano- and Microfluidics at the Technical University of Darmstadt about data-driven reduced-order models for fluid flows.
May 23, 2024: Our article on Global sensitivity analysis with limited data via sparsity-promoting D-MORPH regression: Application to char combustion appeared in the Journal of Computational Physics. Therein, we present a new method that can effectively learn surrogate models from very limited high-fidelity simulations. Those models are then used for Sobol sensitivity analysis to determine influential parameters for subsequent design optimization. Former Postdoc Dongjin Lee and then undergraduate student Elle Lavichant worked on this article.
May 8, 2024: Our joint work Gradient Preserving Operator Inference: Data-Driven Reduced-Order Models for Equations with Gradient Structure with Yuwei Geng, Jasdeep Sing, Lili Ju and Zhu Wang (University of South Carolina) appeared in CMAME. Therein, we extended our previous work on Hamiltonian Operator Inference to systems with more general gradient structure, which also allow for dissipation. The data-driven reduced-order modeling approach is again based on Operator Inference, and provides algorithmic improvements for the Hamiltonian case as well.
April 29, 2024: Our paper on Scalable Computation of Energy Functions for Nonlinear Balanced Truncation (w. Jeff Borggaard, Serkan Gugercin, Linus Balicki) appeard in CMAME. We are interested the problem of nonlinear model reduction via the process of balancing certain energy functions (a.k.a., balanced truncation model reduction). This paper presents a scalable computation of the energy functions via Taylor-series approximations, and Part 2 focuses on reduced-order modeling on the nonlinear balanced manifolds. This is an exciting area of research and has fascinated me for almost a decade now.
April 21-24, 2024: I was honored and look forward to give a keynote at the 9th Thermal and Fluids Engineering Conference organized by the American Society of Thermal and Fluids Engineers (ASTFE), where I presented work on "Data-driven reduced-order modeling for large-scale fluid models".
April 1, 2024: I presented recent work on Scalable Computations for Nonlinear Balanced Truncation Model Reduction in the LSU Control and Optimization Zoom Seminar.
March 8, 2024: We are excited to be part of a Multidisciplinary Research Initiative (MURI) award from AFOSR on the topic of Mathematics of Digital Twins. There are so many interesting topics to work on surrounding reduced-order models and uncertainty quantification to make digital twins be ready to make informed decisions about their physical counterparts. UCSD was part of 4 winning proposals this year, see the news article UC San Diego Among Multidisciplinary Awards Providing $221M Nationally for Cutting-Edge Projects.
February 28, 2024: Postdoc Harsh Sharma's article on Lagrangian operator inference enhanced with structure-preserving machine learning for nonintrusive model reduction of mechanical systems appeared in CMAME today. This is a collaborative work with our colleagues in Structural Engineering, David Najera-Flores and Prof. Michael Todd. In the work, we extended the Lagrangian Operator Inference Method--a method to learn linear ROMs from data of Lagrangian systems with guaranteed structure preservation--to nonlinear systems. We proposed a two-step approach, where the first step learns the linear model structure, and the second step uses a structure-preserving machine learning approach to learn the remaining nonlinear terms. One of the examples uses experimental data from the half Brake-Reuss beam, where we show that all relevant information is accurately reflected in the ROM.
February 27-March 1, 2024: Our group attended the SIAM Uncertainty Quantification in Trieste. I presented Multifidelity Risk Assessment for Nonlinear Systems under High-Dimensional Dependent Random Variables (in MS168: Theory and Simulation of Failure Probabilities and Rare Events) and fourth-year year PhD student Nate Linden will give a talk on Multimodel Inference to Account for Model Uncertainty in Systems Biology (MS203: Mitigating Parametric and Model Uncertainty in Systems Biology) which we are co-organizing with Padmini Rangamani, Daniele Schiavazzi.
January 26, 2024: It was great to speak at the online seminar series Data-Driven Physical Simulation (DDPS) organized by Youngsoo Choi (Lawrence Livermore National Laboratory) on the topic of "Structure-Preserving Learning of High-Dimensional Lagrangian and Hamiltonian Systems". The recording is available on their Youtube Channel here
December 2023: Third-year graduate student Opal Issan started working on spectral methods for simulating plasmas, such as the Vlasov-Poisson equations over the summer with collabortors Alex Koshkarov and Gian Luca Delzanno at Los Alamos National Laboratories and Frederico Halpern at General Atomics. The proposed anti-symmetric spectral discretization preserves the anti-symmetric structure of the advection operator in the Vlasov equation, resulting in a stable numerical method. Moreover, Opal was able to prove many conservation properties of the discretized system. The results of that work are now availabe as a preprint: Anti-symmetric and positivity preserving formulation of a spectral method for Vlasov-Poisson equations.
November 21, 2023: We are very excited to be part of a multidisciplinary team at UCSD that has been awarded a $3.6 million grant from the Air Force Research Laboratory (AFRL) to make the design and risk assessment of next-gen Air Force vehicles more efficient. The six-year grant supports the new AFRL-UC San Diego Collaborative Center for Optimal Risk-quantified and robust Design of aerospace vehicles (ACCORD). The goal of the center is to advance the computational design methods that are crucial for the development of next-generation Air Force vehicles, including electric vertical takeoff and landing (eVTOL) aircraft and supersonic air vehicles. See the for more details announcement.
September 15, 2023: I gave a talk in the Computational Mathematics and Scientific Computing seminar at the Courant Institute on the topic of "Scalable computations for nonlinear balanced truncation model reduction".
September, 2023: Welcome to our new graduate students! Elle Lavichant starts her M.S. in MAE and continues the research she has done as a B.S. student on biomass combustion with us, Steven Nguyen (co-advised with Prof. Jorge Cortes) is joining us from UC Santa Barbara, and Dylan Hirsch (co-advised with Prof. Sylvia Herbert) will be joining us from MIT.
September 8, 2023: Postdoc Harsh Sharma's article on Symplectic model reduction of Hamiltonian systems using data-driven quadratic manifolds got accepted at CMAME. The workcombines the expressivness of quadratic approximation manifolds with projection-based model reduction that enforces symplecticity. This was a large collaboration (with Silke Glas and Hongliang Mu, University of Twente, Patrick Buchfink, U. Stuttgart, Rudy Geelen, UT Austin) and combined expertises in multiple areas.
August 20-25, 2023: Opal Issan and I presented at the 10th International Congress on Industrial and Applied Mathematics (ICIAM 2023) in Tokyo. Together with Yuto Miyatake (Kyoto), we organized a session on Learning Dynamical Systems by Preserving Symmetries, Energies, and Variational Principles and together with Enrico Camporeale (NOAA and UC Boulder) we organized a session on Space Weather: Modeling, Surrogates and Uncertainty Quantification.
August 2-4, 2023: Dongjin Lee and Nate Linden attended the UQ Summer School at USC.
July 24-26, 2023: Nick Corbin and I attended the biannual SIAM Conference on Control and Its Applications in Philadelphia. Together with Jeff Borggaard and Serkan Gugercin, I organized a two-part minisymposium on Nonlinear Model Order Reduction for Control Applications where both Nick and I presented recent work.
July 23-27, 2023: Postdoc Dongjin Lee presented his work at the 17th U. S. National Congress on Computational Mechanics in Albuquerque, NM.
July, 2023: Congratulations to Postdoc Dongjin Lee, who will be starting an Assistant Professor position at Hanyang University (Seoul, Sout Korea) in the Department of Automotive Engineering. Dongjin has research interests in design optimization, uncertainty quantification, and significant work experience with Hyundai Motors.
July 16-21, 2023: Nate Linden presented his work on "Multimodel modeling for blood glucose and insulin measurements in diabetes" at the 2023 Society of Mathematical Biology Annual Meeting at Ohio State University.
July 5, 2023: We collaborated with Ruijian Han, Akil Narayan and Yiming Xu on the paper An approximate control variates approach to multifidelity distribution estimation, which just posted on arxiv. The suggested method can effectively estimate cumulative distribution functions of quantities of interest, which we demonstrated on a two-dimensional fibre-composite material model.
June 24, 2023: Congratulations to PhD student Nate Linden for publishing his second journal paper, this time on Bayesian parameter estimation for the inclusion of uncertainty in progressive damage simulation of composites in the Journal of Composite Structures.
June, 2023: Congratulations to our undergraduate researchers Elle Lavichant (ME) and Hannah Haider (AE) for earning their BS degrees from the Jacobs School of Engineering. Elle will continue in our group in the MS program and Hannah is moving on to Columbia University to start her PhD.
May 22-26, 2023: Together with Profs. Jeff Borggaard and Serkan Gugercin we organized a Workshop and Conference: Nonlinear Model Reduction for Control at Virginia Tech. We had a great week of tutorials and research talks. Participants particularly enjoyed that there was plenty of time for discussion and seeding future collaborations. Thanks also to the NSF-CMMI program for giving us a presentation.
May 13, 2023: Graduate student Opal Issan just posted her second paper Bayesian Inference and Global Sensitivity Analysis for Ambient Solar Wind Prediction on arxiv. In collaboration with Pete Riley (Predictive Sciences) and Enrico Camporeale (NOAA/CU Boulder) Opal proposed a comprehensive uncertainty quantification (UQ) framework that is able to successfully quantify and reduce parametric uncertainties in a chain of models that maps GONG magnetograms to ambient solar wind near Earth. The work involved global sensitivity analysis, Markov chain Monte Carlo methods and in-depth analysis of the model chain. She will present this work at the Geospace Environment Modeling Summer Workshop in San Diego, June 11-16.
May 10, 2023: Congratulations to Opal Issan for having her work appear in a SIAM News Blog post on Speeding Up Solar Wind Forecasts with Reduced-order Modeling.
April 22, 2023: Our group attended the Southern California Applied Mathematics Symposium at UC Irvine. Nate Linden, Dongjin Lee and Harsh Sharma presented their recent research.
April 15, 2023: We participated with a science booth themed "Space Research at Barrio Logan Science & Art Expo, an inclusive science, art, and culture event that welcomes families from around San Diego County. This event features the Southeast San Diego STEM Ecosystem, UC San Diego, and other community partners to highlight science, art, and culture in the world around us. Thanks to Opal Issan, Nate Linden and Nick Corbin for volunteering for the event. As always, it is great to partner with our two UCSD student clubs Students for the Exploration and Development of Space (SEDS) and the Rocket Propulsion Laboratory for this event.
April 6-7, 2023: Opal Issan and Dongjin Lee attended the USACM workshop on establishing benchmarks for data-driven modeling of physical systems at USC. Opal is presenting "Predicting solar wind streams from the inner-heliosphere to earth via shifted operator inference" and Dongjin is presenting "Multifidelity risk assessment for nonlinear systems under high-dimensional dependent random variables."
March 30, 2023: I gave a seminar talk at the School of Aerospace Engineering Seminar Series at Georgia Tech.
March 27-29, 2023: I attended the AAAI Spring Symposium on Computational Approaches to Scientific Discovery in San Francisco, and present a talk on "Learning Exact and Optimal Quadratic Forms for Nonlinear Non-Autonomous ODEs". This was a great way to meet people from different communities working on a central problem in computational science.
March 14, 2023: Congratulations to Nate Linden whose SIAM News Blog post on Identifiability and Sensitivity Analysis for Bayesian Parameter Estimation in Systems Biology appeared today.
March 9-10, 2023: The Space Weather with Quantified Uncertainties Spring Meeting 2023 took place at MIT with a full 2-day program and a trip to MIT Haystack observatory. Graduate student Opal Issan, undergraduate student Hannah Haider, and I attended this well-run NSF program workhop. Opal presented a poster on ``Parameter Estimation of Ambient Solar Wind Models using ACE Observations".
March 4, 2023: As our first science outreach event this year, we hosted a science booth themed "Space Research at UCSD" at the Comienza con un Sueno on UCSD's campus. Thanks to Opal Issan, Nate Linden and Nick Corbin for volunteering for the event. As always, it is great to partner with our two UCSD student clubs Students for the Exploration and Development of Space (SEDS) and the Rocket Propulsion Laboratory for this event.
February 26-March 3, 2023: Postdoc Harsh Sharma and I attended the SIAM Conference on Computational Science and Engineering (CSE23) in Amsterdam. Together with Prof. Gleb Pogudin, we organized an 8-speaker minisymposium on Exact Polynomialization and Quadratization of Nonlinear Dynamics; together with Prof. Silke Glas and Harsh Sharma we organized an 8-speaker minisymposium on Structure-Preserving Model Reduction for Lagrangian and Hamiltonian Systems; and I presented work (with Opal Issan) on The Shifted Operator Inference Method for Learning Solar Wind Models. SIAM CSE was a well-run and once again fantastic event for our community.
February 6-February 24, 2023: I was in residence at the Isaac Newton Institute at the University of Cambridge, UK, for the semester program on The mathematical and statistical foundation of future data-driven engineering, specifically the deep-dive session on Optimal Control and Inference organized by Susana Gomes and Sebastian Reich. It was a productive three weeks with many interesting discussions.
February 7, 2023: At long last, the second part to our paper series, Nonlinear Balanced Truncation: Part 2-Model Reduction on Manifolds. (with Jeff Borggaard and Serkan Gugercin) posted on arxiv. In the work, we leverage the energy functions of nonlinear dynamical systems (computed in Nonlinear Balanced Truncation: Part 1-Computing Energy Functions) and proposes a strategy to simulataneously balance and reduce the nonlinear systems. The nonlinear coordinate transformation to achieve this is of polynomial form and defines a balanced approximate nonlinear manifold where the system is reduced on. .
February 1, 2023: Our paper Physics-informed regularization and structure preservation for learning stable reduced models from data with operator inference. (with Nihar Sawant and Benjamin Peherstorfer) appeared in CMAME. The paper shows how to enforce stability of ROMs in the operator inference procedure, and uses key results from Stability Domains for Quadratic-Bilinear Reduced-Order Models..
February 1, 2023: Congratulations to Postdoc Dongjin Lee for publishing his first article as a member of my group: Bi-fidelity conditional value-at-risk estimation by dimensionally decomposed generalized polynomial chaos expansion in Structural and Multidisciplinary Optimization. Risk measure estimation has been an interest of our group for a few years now, and this work addresses a specific challenge of high-dimensional and dependent input random variables. Most existing methods make an independence assumption, yet Dongjin's dynamically decomposed generalized polynomial chaos expansion (DD-GPCE) can handle arbitrary and dependent input RVs. Moreover, we suggest an additional approximation of the output quantity of interest via a Fourier polynomial expansion, reducing computational cost of the estimation process when we are dealing with complex systems with large state spaces.
February 1, 2023: I gave a seminar talk at the School of Nuclear Science & Engineering at Oregon State University.
December 8, 2022: I gave a talk at the SimTech Seminar on Model Reduction and Data Techniques for Surrogate Modelling at the the University of Stuttgart, Germany, about "Learning dynamics of nonlinear systems via structure-preserving operator inference".
December 6-9, 2022: Postdoc Harsh Sharma attended the 61st IEEE Conference on Decision and Control and presented his paper Bayesian Identification of Nonseparable Hamiltonian Systems Using Stochastic Dynamic Models (joint work with collaborators Nicholas Galioto and Alex A. Gorodetsky at University of Michigan). In this paper, we propose a probabilistic Bayesian formulation for system identification (ID) and estimation of nonseparable Hamiltonian systems using stochastic dynamic models.
November 7, 2022: Congratulations to graduate student Opal Issan for having her first journal paper appear at the Journal of Computational Physics (free link until Dec 27). With a passion for space weather models, Opal focuses on a background solar wind model and proposed shifted Operator Inference (sOPINF), which extends the existing OPINF learning framework to (a) automatically detect translational shifts (advection) in the data and subsequently removes them as a preprocessing step, and (b) learns and simulates ROMs in the advection-free coordinates, where much lower-dimensional models are possible. She tested that framework on three different solar wind models and got nice results that should aid us in uncertainty quantification later on.
November 2, 2022: Congratulations to graduate student Nate Linden for having his first journal paper appear at PLOS Computational Biology (link). The paper proposed a comprehensive framework for Bayesian parameter estimation and complete quantification of the effects of uncertainties in the data and models. We applied these methods to a series of signaling models of increasing mathematical complexity. The results highlighted how focused uncertainty quantification can enrich systems biology modeling and enable additional quantitative analyses for parameter estimation.
October 14, 2022: It was exciting to return to my alma mater Virginia Tech to give a colloqium talk in the Department of Mathematics about Learning of Structured Dynamical Systems from Data.
September 28, 2022: Problems that politics is dealing with can be approached from an engineering perspective! It is common knowledge that the US Electoral College and the Electoral Count Act need reforms. Together with Chris Truax, a member of the Guardrails of Democracy Project we developed a novel way of informing policy makers how to make better decisions, regardless of party affiliation. This non-partisan, quantitiative engineering solution is based on Monte Carlo simulation and essentially computes the probabilities that the Electoral College and the Electoral Count Act produces the correct president. A sneak-peak of our findings is published in the Bulwark under The Electoral Count Act Is Actually an Engineering Problem. This would have not been possible without Jonathan Carreon, a BS/MS student in applied mathematics and aerospace engineering. More details will follow in the coming year.
September 26-30, 2022: The SIAM MDS was in San Diego, so there was a lot of activity from our group. Together with Dr. Christine Allen-Blanchette we are hosting two minisymposia MS14 & MS 31: Learning Dynamical Systems by Preserving Symmetries, Energies, and Variational Principles where Dr. Harsh Sharma is also presenting his work Preserving Lagrangian Structure in Data-Driven Reduced-Order Modeling of Large-Scale Mechanical Systems. Moreover, Nick Galioto is presenting our collaborative work on Learning Partially Observed Stochastic Dynamical Systems. Graduate student Opal Issan presents her work on Predicting Solar Wind Streams from the Inner-Heliosphere to Earth via Shifted Operator Inference. Then, together with Prof. Padmini Rangamani and our co-advised graduate student Nate Linden we are hosting two minisymposia on MS110 & MS126 Parameter Inference and Uncertainty Quantification for Systems Biology and Medicine where Nate presents his work A Framework for Bayesian Parameter Estimation and Uncertainty Quantification in Systems Biology. Lastly, Postdoc Harsh Sharma is organizing MS146: Exploiting Hamiltonian Structure in Learning Dynamical System Models for Prediction and Control where I am also giving a talk on Hamiltonian Operator Inference: Physics-Preserving Learning of Reduced-Order Models for Canonical Hamiltonian Systems.
September 19-23, 2022: I was very honored to be an invited plenary speaker at the Model Reduction and Surrogate Modeling (MORE) conference in Berlin. The MORE is merger of the triannual MoRePaS and MODRED conferences, and the leading conference of the model reduction community.
September 19, 2022: Our paper Bayesian Identification of Nonseparable Hamiltonian Systems Using Stochastic Dynamic Models (with Postdoc Harsh Sharma and collaborators Nicholas Galioto and Alex A. Gorodetsky at University of Michigan) posted on arxiv and is accepted for publication at the 61st IEEE Conference on Decision and Control (CDC), December 6-9. In this paper, we propose a probabilistic Bayesian formulation for system identification (ID) and estimation of nonseparable Hamiltonian systems using stochastic dynamic models.
August 18-19, 2022: Postdocs Harsh Sharma and Dongjin Lee attended the USACM Thematic Conference on Uncertainty Quantification for Machine Learning Integrated Physics Modeling (UQ-MLIP) and presented posters on Bayesian Identification of Nonseparable Hamiltonian Systems Using Stochastic Dynamic Models (H. Sharma) and A Bi-Fidelity Method for Coherent Risk Assessment of Non-Linear Systems Under Dependent and High-Dimensional Random Variables (D. Lee).
August 13, 2022: I wrote a short News & Views article for Nature Computational Science about Learning state variables for physical systems. The problem of automatically determining state variables for physical systems is challenging, but essential in the modeling process of almost all scientific and engineering processes. That short perspective/discussion article reviews recent work and highlights a few interesting directions.
August 13, 2022: In our third science outreach event this year, we hosted a science booth themed "Space Research at UCSD" at the Southeast San Diego Science & Art Expo, an inclusive science, art, and culture event that welcomes families from around San Diego County. This event features the Southeast San Diego STEM Ecosystem, UC San Diego, and other community partners to highlight science, art, and culture in the world around us.
August 10, 2022: Welcome to our new PhD students Hyeonghun Kim and Boyang Li. Hyeonghun is interested in data-driven reduced-order modeling methods to better understand high-dimensional dynamical systems and to control them. He joins us with a B.S. in Mechanical & Electrical Control Engineering from Handong Global University in South Korea. Boyang is co-advised with Prof. Sylvia Herbert and is interested in developing control methodologies that possess both mathematical formality and versatility in application to various robotics systems, drawing ideas from optimization theory, dynamical systems, and machine learning. Boyang just graduated with a B.Sc. in Mathematics and Physics from William & Mary.
June 19 - July 02, 2022: Opal Issan and Boris Kramer attended the back-to-back Geospace Environment Modeling (GEM) workshop and the Solar Heliospheric and INterplanetary Environment (SHINE) workshop and present their work on reduced-order modeling for solar physics applications there. This is a new research direction for our group and is funded through the NSF SWQU: Composable Next Generation Software Framework for Space Weather Data Assimilation and Uncertainty Quantification. We learned a good deal about this application and saw some really exciting research.
July 31-August 5, 2022: The WCCM was moved to fully virtual. We organized a minisymposium together with Yuto Miyatake on Structure-preserving model reduction for nonlinear systems.
June 1-3, 2022: To honor Prof. Terry Herdman's retirement from Virginia Tech, there was a conference on Applied and Computational Mathematics. where I could give a talk on "Learning reduced-order models via structure-preserving operator inference". It was great to be back to Virginia Tech and see all the great things the ``ICAMlers" have done through the decades..
May 31, 2022: Our research group attended the Southern California Applied Mathematics Symposium (SOCAMS) sponsored by SIAM and hosted by Harvey Mudd. They presented their most recent research as follows: Dr. Harsh Sharma ("Preserving Lagrangian Structure in Data-Driven Reduced-Order Modeling of Large-Scale Mechanical Systems"), Opal Issan ("Predicting Solar Wind Streams from the Inner-Heliosphere to Earth via Shifted Operator Inference"), Dr. Dongjin Lee ("A bi-fidelity method for conditional-value-at-risk estimation under dependent and high-dimensional random variables"), Nate Linden ("A Bayesian Framework for Parameter Estimation from Sparse and Noisy Measurement Data in Systems Biology"). This was a great opportunity to meet researchers and graduate students in the Southern California region working on similar problems.
May 23-June 01, 2022: The Institute for Computational and Experimental Research in Mathematics (ICERM) at Brown University is doing a fantastic job of hosting the Spring 2020 Reunion Event. This event gets researchers from the winter/spring 2020 workshops back together in person. It was a great time to see many of my colleagues again and to work together on publications, proposals, and just to discuss research.
April 12-15, 2022: It was great to attend the SIAM UQ 2022 conference in Atlanta in person again and seeing so many familiar faces. Together with Prof. Rebecca Morrison, we organized a minisymposium with seven speakers on Uncertainty quantification and data assimilation for space weather applications, which highlighted research on our project SWQU: Composable Next Generation Software Framework for Space Weather Data Assimilation and Uncertainty Quantification. Postdoc Dr. Dongjin Lee presented his recent work Coherent Risk Assessment for Nonlinear Structural Analysis via Reduced-Order Models and graduate student Nate Linden presented his work on Bayesian Parameter Estimation from Sparse and Noisy Measurement Data in Systems Biology.
April 12, 2022: Nate Linden's first paper since joining UCSD just posted. Its title is Bayesian Parameter Estimation for Dynamical Models in Systems Biology. The paper is addressing the challenges that one faces with UQ in systems biology models, which are low-dimensional (few states) but have a much higher number of parameters that enter nonlinearly into the ODEs. Plus, one is almost never able to sense all state variables, and the measurements are sparse in time and corrupted with noise. Nate proposes a comprehensive framework that starts with identifiability and sensitivity analysis to reduce the number of parameters to be learned, and then uses the unscented Kalman filter Markov chain Monte Carlo algorithm to learn the posterior distribtions of the parameters.
April 7, 2022: Postdoc Dongjin Lee posted his recent work Bi-fidelity conditional-value-at-risk estimation by dimensionally decomposed generalized polynomial chaos expansion. on arxiv. Risk measure estimation has been an interest of our group for a few years now, and this work addresses a specific challenge of high-dimensional and dependent input random variables. Most existing methods make an independence assumption, yet Dongjin's dynamically decomposed generalized polynomial chaos expansion (DD-GPCE) can handle arbitrary and dependent input RVs. Moreover, we suggest an additional approximation of the output quantity of interest via a Fourier polynomial expansion, reducing computational cost of the estimation process when we are dealing with complex systems with large state spaces.
Mar 28, 2022: Opal Issan's paper Predicting solar wind streams from the inner-Heliosphere to Earth via shifted operator inference. is up on arxiv. The work derives novel ROMs to predict solar wind from the heliosphere to Earth and compares the derived models to reduced-physics approximations, finding that the latter are less accurate. Along the way, Opal derived a new shifted operator inference framework to learn polynomial dynamical systems models directly from data by incorporating translational symmetries into the model learning method.
Mar 12, 2022: Harsh Sharma's paper Preserving Lagrangian structure in data-driven reduced-order modeling of large-scale mechanical systems. is up on arxiv. This work presents a nonintrusive physics-preserving method to learn reduced-order models for Lagrangian mechanical systems. The numerical results demonstrate Lagrangian operator inference on an Euler-Bernoulli beam model and a soft-robotic fishtail model with 779,232 degrees of freedom.
Feb 4, 2022: Upon placing 49 new Starlink satellites in low-earth orbit, the following day 40 of those were lost due to a geomagnetic storm that caused atmospheric drag to increase by as much as 50%, see some references here and at space.com. This shows the importance of predicting space weather events and atmospheric drag. Our project SWQU: Composable Next Generation Software Framework for Space Weather Data Assimilation and Uncertainty Quantification is working on exactly that, as are integrating data-driven and projection-based model reduction for space weather and use them in the context of uncertainty quantification.
Jan 18, 2022: I am giving a talk about Learning dynamics of nonlinear fluid models via structure-preserving operator inference at the fluid mechanics seminar at Stanford University.
Jan 11, 2022: I gave a virtual talk about Learning dynamics of nonlinear fluid models via structure-preserving operator inference at the numerical optimization seminar at University of Konstanz, Germany.
Jan 05, 2022: Very honored to have won an NSF CAREER award for my project Goal-Oriented Variable Transformations for Efficient Reduced-Order and Data-Driven Modeling.
Jan 01, 2022: I am very excited to have been awarded a new NSF project on Nonlinear Balancing: Reduced Models and Control together with my colleagues Serkan Gugercin and Jeff Borggaard at Virginia Tech.
Dec 2021: Postdoc Harsh Sharma's paper Hamiltonian operator inference: physics-preserving learning of reduced-order models for Hamiltonian systems. (in collaboration with Zhu Wang) posted online at Physica D: Nonlinear Phenomena. The paper shows how to learn reduced-order models for Hamiltonian systems from data only, and demonstrates this on a variety of nonlinear Hamiltonian models..
Dec 2021: Our paper Certifiable risk-based engineering design optimization (with Anirban Chaudhuri, Matthew Norton, Johannes Royset and Karen Willcox) posted online at AIAA Journal. The paper shows that coherent and regular risk measures have many advantages compared to using reliability in design optimization, such as they preserve convexity of the limit state function, and provide data-driven conservativeness for the ensuing designs.
Nov 15, 2021: I gave a talk on "Multifidelity modeling for engineering design with coherent risk measures" at Sandia National Labs UQ semniar series.
July 2021: Our paper Physics-informed regularization and structure preservation for learning stable reduced models from data with operator inference. (Nihar Sawant and Benjamin Peherstorfer) just posted on arxiv. The paper shows how to enforce stability of ROMs in the operator inference procedure, and uses key results from Stability Domains for Quadratic-Bilinear Reduced-Order Models..
Feb. 2021: Science News published an article on Solar storms can wreak havoc. We need better space weather forecasts which mentiones our work in space weather modeling.